Speakers
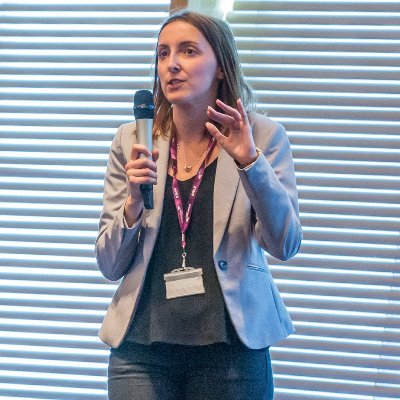
NLG for Human-Robot Interaction: Challenges and Opportunities
Abstract:Human-robot interaction (HRI) focuses on researching the interaction between humans and (mostly) physical robots. Despite the media coverage of robots displaying human-level capabilities in conversational dialogue and NLG, in reality, such robots use simple template-based approaches and follow pre-scripted interactions. In this talk, I will initially provide an overview of current approaches to NLG for HRI focusing on the limitations of current approaches and emphasising the challenges of developing flexible NLG approaches for HRI. Finally, I will provide an overview of our project CiViL: Commonsense and Visually-enhanced natural Language generation and discuss future directions.
Bio: Dimitra Gkatzia is an Associate Professor at the School of Computing at Edinburgh Napier University and a SICSA AI Theme co-lead. Dimitra is interested in making computers and robots interact in a human-like way using natural language, while at the same time respecting the privacy of the users. Her current work on Human-Robot Interaction focuses on the interplay between various modalities (vision, speech, knowledge-bases) in real-world settings for human-robot teaming scenarios. Her work in this area focuses on enhancing computers/robots' conversational capabilities with 'commensense' similar to the ones present in human-human communication. She is also interested in NLG evaluation practices as well as addressing the challenges of NLG in low-resource settings where example relevant tools are scarce and data is not freely available, or it is hard to acquire due to challenges such as privacy issues or low numbers of native speakers of a language.
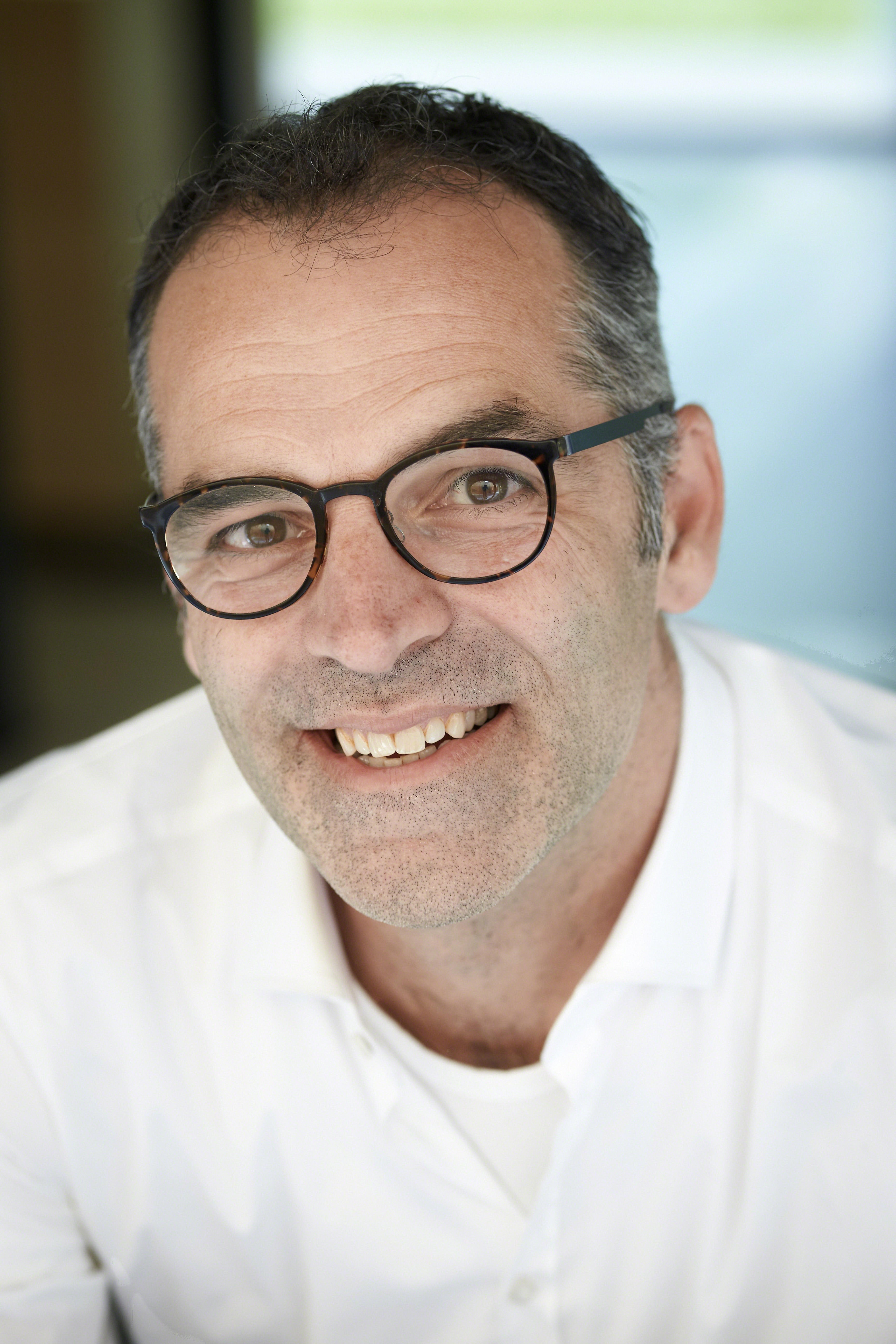
From Generating Personalised Risk Descriptions to Data-driven Health Narratives: Applying NLG to Healthcare Data
Abstract:Even though the health domain has been mentioned as a potential application domain for NLG since the early days of the field, one thing that has changed recently is the emergence of large and growing amounts of patient-generated health data in hospitals and other care facilities (think of self-reported outcome measures, electronic health records, registry data). Hospitals are urgently looking for ways to make this data accessible in a personalised manner for both patients and clinicians. Naturally, this is a task for which NLG lends itself very well. In this talk, I describe how traditional NLG tasks, such as the generation of referring expressions and of narratives, re-emerge in this health domain. I also highlight which particular evaluation questions this raises, such as, for example, what kind of information do patients actually want? In which format do they prefer this information? What cognitive implications does access to this information have? In the final part of the talk I touch upon a number of broader questions, such as is there room for transformers in this application domain, and what are ethical and privacy issues for this kind of application.
Bio: Prof. Dr. Emiel Krahmer is a full professor in the Tilburg School of Humanities and Digital Sciences. In his research, he studies how people communicate with each other, and how computers can be taught to communicate in a similar fashion, to improve communication between humans and machines. His current research is positioned at the intersection of artificial intelligence, natural language processing and human communication studies, and has applications in, for example, media (e.g., automatic moderation and summarization of online discussions), health (e.g., data-driven treatment decision aids; chatbots for smoking cessation) and education (e.g., social robots teaching children a second language).
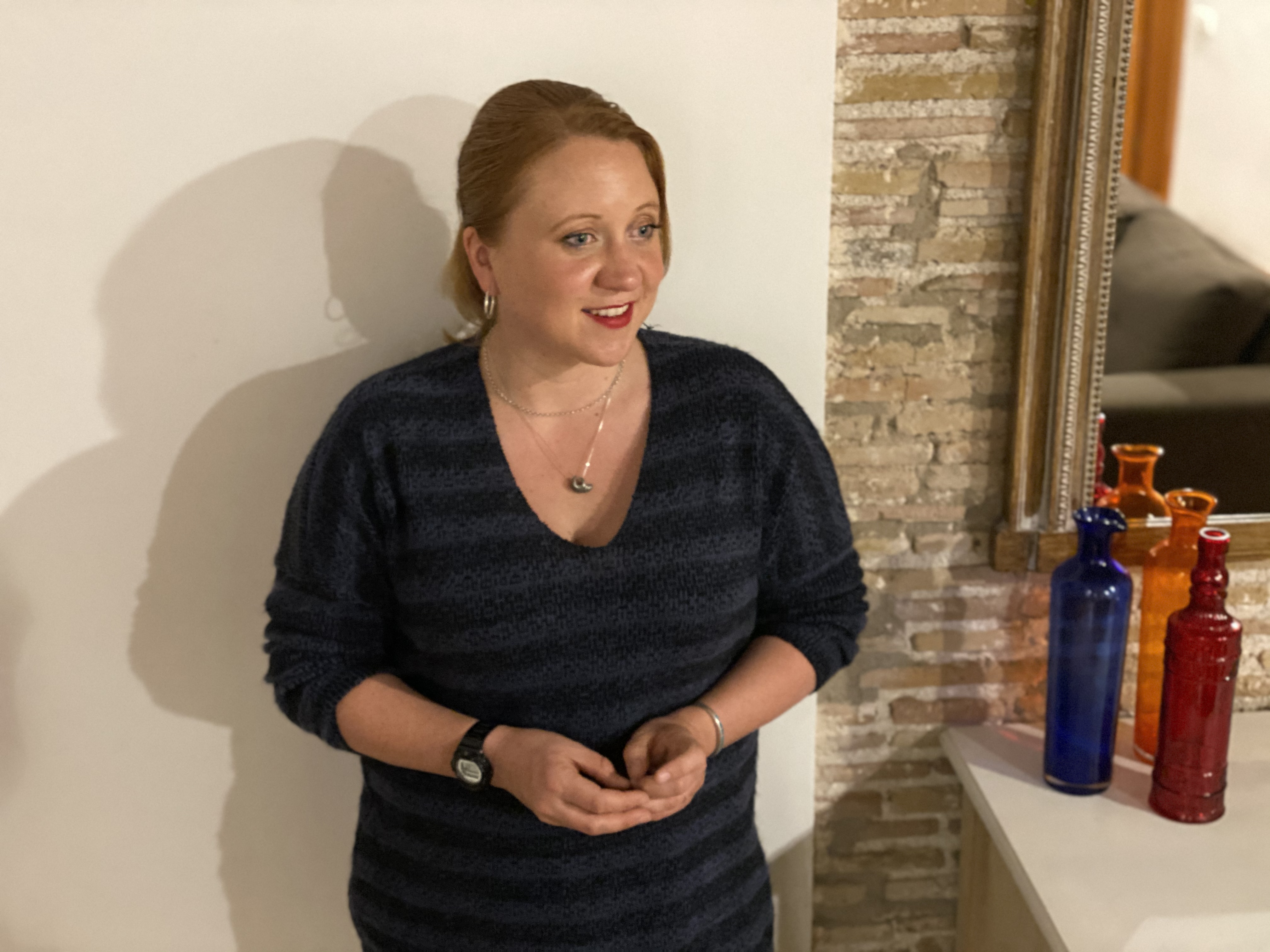
Advancing Natural Language Generation (for better or worse)
Bio: Margaret Mitchell is a researcher working on Ethical AI, currently focused on the ins and outs of ethics-informed AI development in tech. She has published over 50 papers on natural language generation, assistive technology, computer vision, and AI ethics, and holds multiple patents in the areas of conversation generation and sentiment classification. She currently works at Hugging Face driving forward work in the ML development ecosystem, ML data governance, AI evaluation, and AI ethics. She previously worked at Google AI as a Staff Research Scientist, where she founded and co-led Google's Ethical AI group, focused on foundational AI ethics research and operationalizing AI ethics Google-internally. Before joining Google, she was a researcher at Microsoft Research, focused on computer vision-to-language generation; and was a postdoc at Johns Hopkins, focused on Bayesian modeling and information extraction. She holds a PhD in Computer Science from the University of Aberdeen and a Master's in computational linguistics from the University of Washington. While earning her degrees, she also worked from 2005-2012 on machine learning, neurological disorders, and assistive technology at Oregon Health and Science University. She has spearheaded a number of workshops and initiatives at the intersections of diversity, inclusion, computer science, and ethics. Her work has received awards from Secretary of Defense Ash Carter and the American Foundation for the Blind, and has been implemented by multiple technology companies. She likes gardening, dogs, and cats.
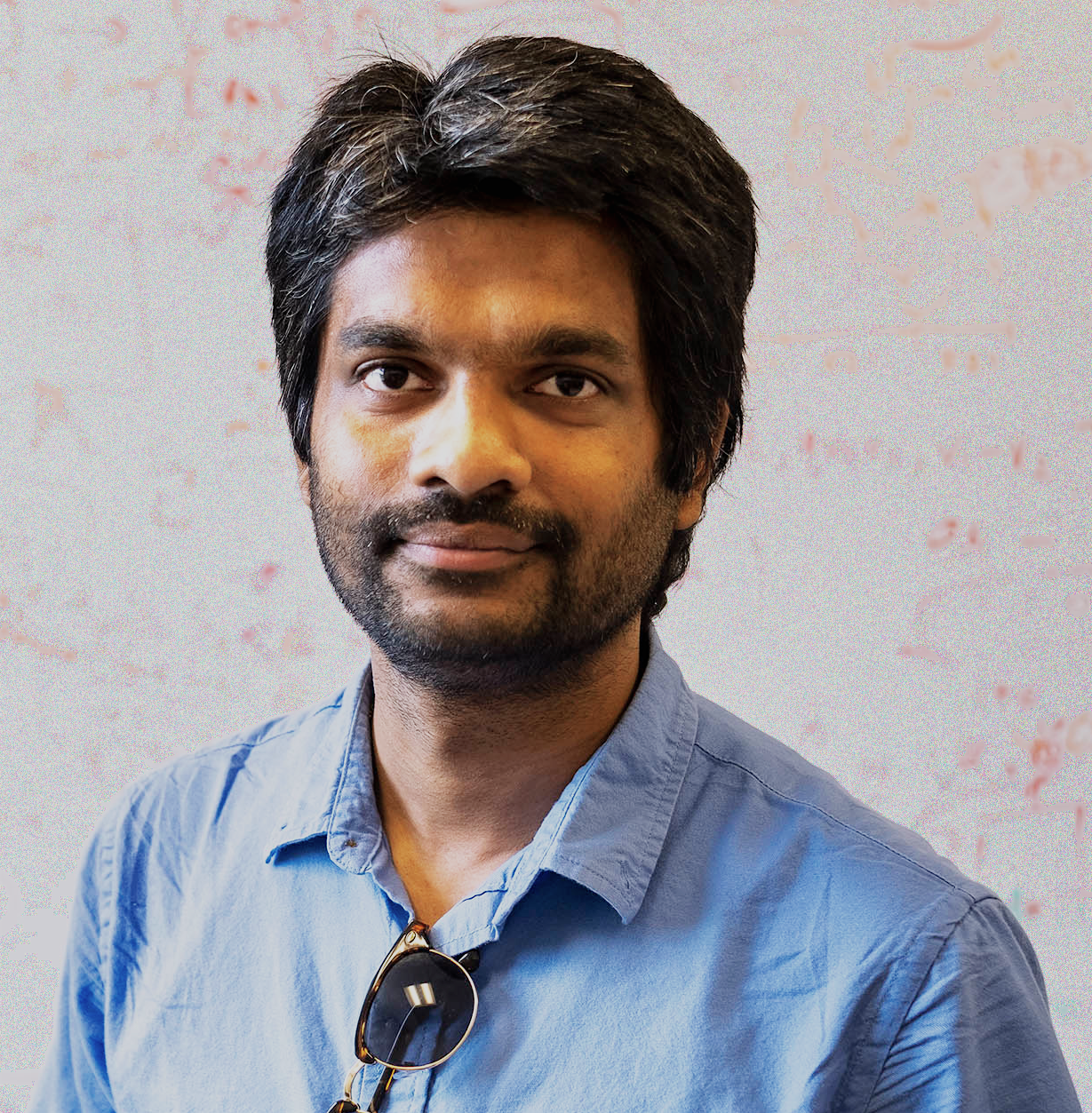
Modeling and Evaluating Faithful Generation in Language and Vision
Abstract:Faithfulness is a key aspect of accurate and trustworthy generation in diverse modalities such as language and vision. In this talk, I will present work towards modeling and evaluating faithfulness in summarization and multimodal tasks. First, we will discuss our earlier work on multi-task and reinforcement learning methods to incorporate auxiliary faithfulness-promoting skills such as entailment and back-translation validity. We will then describe abstractive summarization models that holistically address the problem of faithfulness during pre-training and fine-tuning. Next, we will explore improved summary faithfulness evaluation methods based on human-automation balance and semantic graph representations. Lastly, we will briefly discuss faithful, fine-grained skill evaluation of text-to-image generation models.
Bio: Dr. Mohit Bansal is the John R. & Louise S. Parker Professor in the Computer Science department at University of North Carolina (UNC) Chapel Hill. He received his PhD from UC Berkeley and his BTech from IIT Kanpur. His research expertise is in natural language processing and multimodal machine learning, with a particular focus on grounded and embodied semantics, human-like language generation and Q&A/dialogue, and interpretable and generalizable deep learning. He is a recipient of the DARPA Director's Fellowship, NSF CAREER Award, and Army Young Investigator Award. His service includes ACL Executive Committee, ACM Doctoral Dissertation Award Committee, Program Co-Chair for CoNLL 2019, ACL Americas Sponsorship Co-Chair, and Associate/Action Editor for TACL, CL, IEEE/ACM TASLP, and CSL journals.